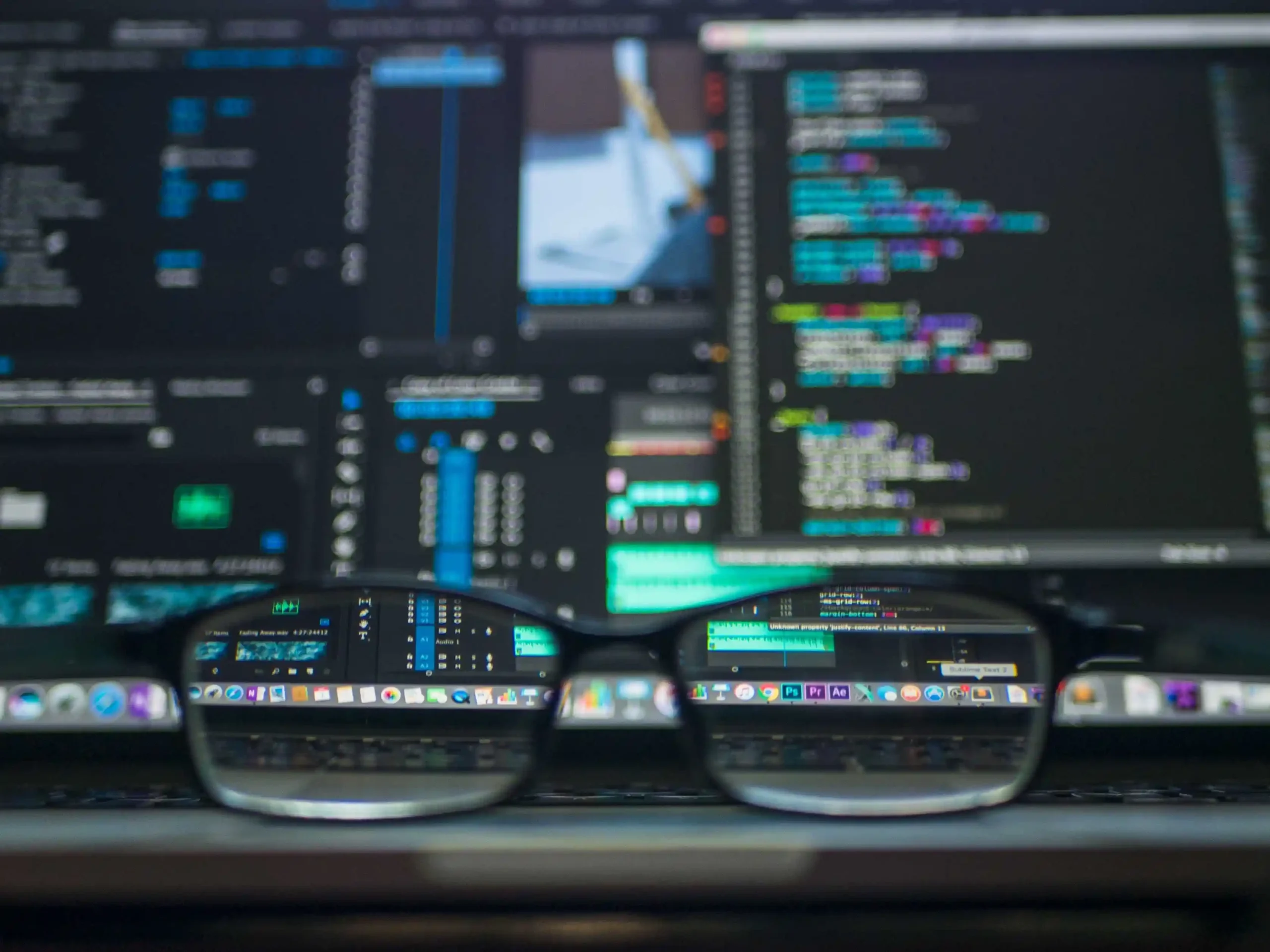
Today, deep learning is at the forefront of rapid advances in artificial intelligence, a powerful technology that enables computer systems to solve complex tasks in a human-like manner. Deep learning is a sub-branch of machine learning that involves the ability to recognize patterns in large data sets and perform complex tasks.
Deep learning is based on biological neural networks and aims to train computer systems by creating artificial neural networks. As such, deep learning models perform learning through a network structure consisting of several layers. Each layer learns more complex features and patterns by processing input data. So, what is the difference between deep learning and machine learning? What are the use cases of deep learning? Let’s take a look at our guide!
Application Areas of Deep Learning
Deep learning has a wide range of applications and is used in many industries and sectors. Deep learning models are successfully applied in many areas such as automatic recognition, natural language processing, image and voice analysis.
Image Recognition and Classification
Deep learning has achieved great success in image recognition and classification. Many image processing applications use deep learning models for tasks such as object recognition, face recognition and search.
Natural Language Processing (NLP)
Deep learning is used in the field of natural language processing to enhance language understanding and generation capabilities. Deep learning techniques have made a huge impact on tasks such as speech recognition, text translation and sentiment analysis.
Automated Driving Technologies
In the automotive sector, deep learning models are used to perceive the environment of self-driving cars and to act safely. In this way, vehicles can adapt to various traffic situations.
Deep Learning Challenges and Future Perspectives
While deep learning has achieved great success, it also faces some challenges. Issues such as data abundance, computational power and model interpretability are issues that require further development of deep learning systems. In addition, reliability and ethical issues are also important to consider.
In the future, deep learning technology is expected to be further refined and optimized. Developments such as more effective learning with smaller data sets, transfer learning and a focus on industry-specific solutions will contribute to the widespread adoption of deep learning. Deep learning is a powerful technology that has revolutionized the field of artificial intelligence. With evolving algorithms and increasing computational power, deep learning models will continue to shape future technological developments by demonstrating human-like performance on many complex tasks.
What is Deep Learning?
Deep learning is a sub-branch of machine learning that focuses on information extraction and learning capabilities through complex mathematical models such as artificial neural networks. This technology is characterized by the use of neural networks with a multi-layered structure. Deep learning is used to identify patterns from large data sets and perform complex tasks. It is an effective tool for processing and understanding various types of data such as audio, images and text.
What is a Deep Learning Model?
A deep learning model is an artificial intelligence model consisting of multilayer neural networks. These models perform a learning process by analyzing data sets and then applying this learned knowledge to new data. Deep learning models are particularly good at extracting complex patterns from large data sets and are often used in areas such as image recognition, natural language processing and game strategies.
What is Deep Learning and How to Use It?
Deep learning is realized through multilayer neural networks that are trained with many predetermined architectures and parameters. In the training phase, the model is fed with a large set of labeled data and can make future predictions based on patterns learned from this data set. Users can use deep learning models to develop custom applications, automate complex tasks or perform various data analysis tasks. This answers the question of how deep learning works.
What are the Types of Deep Learning?
Deep learning algorithms cover a field that includes various types. Convolutional Neural Networks (CNNs) excel at visual tasks such as image recognition. Recurrent Neural Networks (Recurrent Neural Networks or RNNs) are used to deal with time-dependent data such as time series data. Deep Belief Networks are used for tasks such as feature extraction and classification. These types are designed to meet specific needs for different application areas of deep learning.